A dynamic duo: How machine learning is helping make new discoveries in polymer chemistry
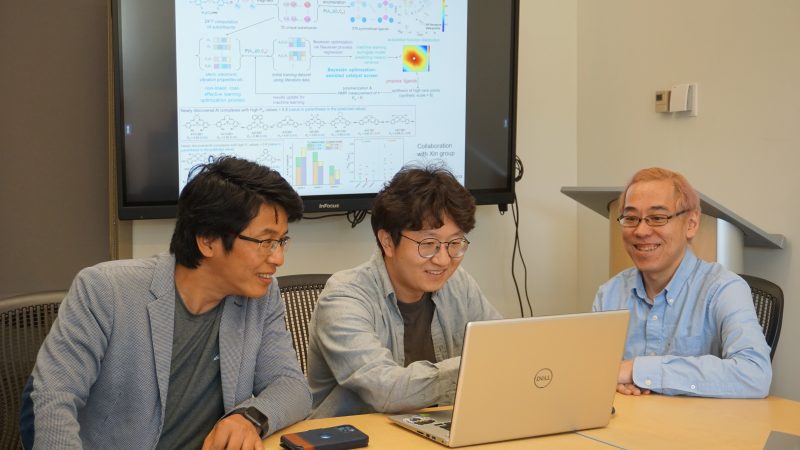
Using a highly efficient algorithm developed by machine learning, Virginia Tech researchers have produced and identified undiscovered catalyst reactions that can help create more sustainable polymers.
Led by Professors Rong Tong and Hongliang Xin, research groups affiliated with the Virginia Tech Macromolecules Innovation Institute overcame the challenge of a small data set to apply artificial intelligence to polymer chemistry. Their research was featured this summer in Nature Communications.
“Visualization can be important for chemistry. Researchers often look at structures in reactions and postulate particular pathways for the reaction. We aim to develop a fine approach to help chemists efficiently find and discover high performance catalysts for polymer reactions,” said Tong, associate professor in the Department of Chemical Engineering and member of the institute.
Their research involves polylactic acids, used frequently as biodegradable materials in agriculture and packaging, and in the medical field as biocompatible/absorbable tissue implants and sutures. They are one of the few bio-sourced, degradable polymers that are commercially produced and approved by the Food and Drug Administration.
Data science can help guide, design, and accelerate the discovery of new catalysts for polymer reactions. But researchers required a highly efficient algorithm that could handle a small-sized data set.
“This is different from typical organic chemistry, where a catalyst can be used for over 1,000 different reactions. That large data set is easy for machine learning to start with,” said Tong. “In polymer chemistry, however, the monomer and polymer are fixed, with less than 100 unique reactions. With such a small database, it is difficult to use common machine learning techniques to model the polymer catalyst system."
As a result, researchers incorporated Bayesian learning, a probablistic machine learning approach, which enabled the algorithm to make effective predications and guide the catalytic materials design, said Xin, associate professor in chemical engineering.
In total, 33 new catalysts were synthesized according to the algorithm and 13 were found to be highly efficient.
Using these new catalysts that promote molecular precision along the chains, researchers can produce degradable polylactic acid with improved thermal and mechanical properties compared to current commercial polylactic acid.
"The integration of AI and machine learning, as demonstrated in this study, paves the way for a more sustainable circular economy by expediting the development and optimization of recyclable and eco-friendly materials,” said Xin.
The research team hopes the findings and methods will benefit the broader polymer chemistry field and advance the development of valuable polymers.
“Catalyst development has long been regarded as a complex and difficult process in polymer chemistry. It is very resource and time extensive,” said Tong. “Using the platform we have provided, this can help many more polymer chemists uncover more catalysts for their reactions."